Technology Disruption: 2015–2025
In 2013, McKinsey published a landmark report identifying the twelve most disruptive technologies expected to reshape the global economy. The report was based on evaluating more than 100 emerging technologies, and each of the final twelve selections was chosen for its high potential to create widespread economic impact. Among these technologies were mobile computing and cloud/SaaS platforms – two innovations that have since become foundational to how we live and work. They reshaped industries, streamlined global commerce, and fueled explosive GDP growth.
Contrast that with knowledge worker automation (KWA) which, of the twelve selected technologies, was projected to be the second most disruptive. While mobile and cloud took off, KWA has vastly underperformed expectations. The McKinsey report projected KWA would deliver between $5.5 trillion and $7.4 trillion in global GDP impact by 2025, including $1.5 to $2 trillion in the U.S. alone. Today, with just months left in that forecast window, estimates suggest the actual GDP impact is closer to $50 billion.
Why Has Knowledge Work Automation Lagged?
Let’s take a minute to better understand the potential scope of automating the knowledge worker. There are currently about 130 million workers in the US, of which roughly 100 million are considered knowledge workers, where the definition for knowledge worker is someone who primarily thinks for a living. These occupations include software developers & engineers, accountants & auditors, lawyers & paralegals, loan officers & underwriters, and teachers & professors.
Despite its promise, knowledge worker automation struggled to gain traction. The problem wasn’t a lack of need or imagination – it was primarily that the technology simply wasn’t ready. Early automation systems like RPA handled rule-based tasks well but failed at the context-rich, language-dependent, nuanced nature of knowledge work.
The real inflection point came with the recent development in 2023-2024 of transformer-based architecture and large language models (LLMs) like GPT-3 and GPT-4. These tools gave machines the ability to interact with human language in a way that was fluid, contextual, and surprisingly nuanced. For the first time, machines could “understand” human queries – and humans could communicate with machines in natural language, without a programmer or business analyst acting as a go-between.
In theory, this means that all knowledge workers can now engage directly with intelligent LLM and GenAI systems using natural language – no coding or technical fluency required. It’s as if each worker suddenly has access to a capable, junior virtual assistant that can help think, write, and communicate. Of course, like any junior assistant, it still needs guidance and oversight.
We’ve officially crossed the tipping point. The way humans interact with machines has fundamentally shifted. Instead of building custom workflows or writing code, you can now get value from intelligent systems simply by asking – just like you would a colleague.
The GenAI Explosion
We’ve seen a phase of rapid adoption and exponential growth of Generative AI over the last two years. GenAI tools are now in the hands of tens of millions of users. People are using it personally, professionally, casually, and strategically. Most importantly, the adoption is bottom-up; it’s not typically CIOs or innovation teams leading the charge. It’s everyday knowledge workers who discover these tools, apply them, and become more productive.
This bottom-up adoption model also explains why the economic impact hasn’t yet registered as traditional GDP growth. GenAI isn’t primarily sold as a platform to enterprises. Instead, it’s saving time and energy for millions of individuals.
Assuming about 30% of the 100 million U.S.- based knowledge workers saw a 15% productivity boost from GenAI technology, which is consistent with current estimates, the projected annual uplift in economic output would reach between $350 and $400 billion. This is only about 20%-25% of the projected $1.5-$2.0 trillion of additional US worker productivity that was forecast for 2025. Again, the anticipated growth in KWA adoption and productivity was likely postponed by several years, as the underlying technology simply wasn’t ready to handle the demands of cognitive intelligence until recently.
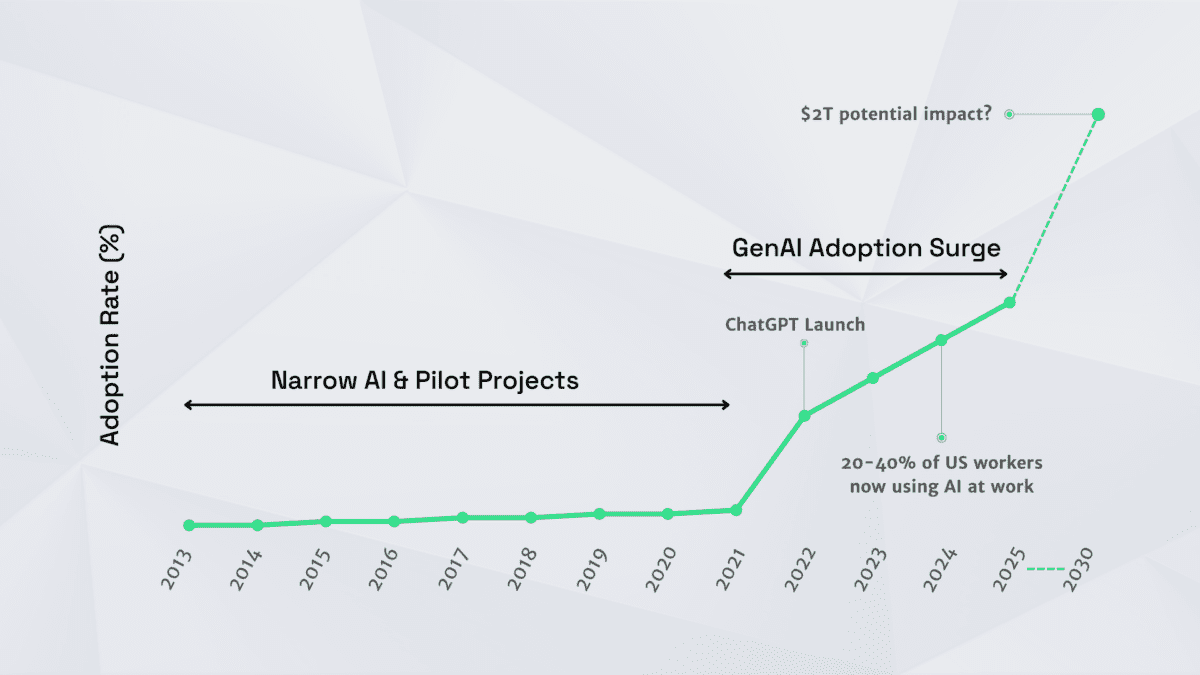
So, we’re not quite there yet – but we’re far closer than ever before. The S-curve has started.
Why Is GenAI Adoption Bottom-Up
GenAI is remarkably capable, but it still needs “adult supervision” in solving complex cognitive tasks. Users often treat it like a junior assistant: “Write me a summary,” or “Draft an outline I can polish.” And it performs, usually quite impressively. But users also know it might hallucinate, miss a detail, or misunderstand intent. So, they check the output. They verify correctness.
With the nature of GenAI being so nondeterministic, it has been hard for executives to harness its potential at the company-wide level. I think many executives are waiting for GenAI to become more reliably predictable in its performance before mandating its use from the top down.
ChatGPT and its competitors are also horizontal tools, not built for specific verticals. This makes it perfect for B2C user adoption, but when it comes to enterprise B2B, many executives are looking for solutions that are more neatly packaged and tailored to their vertical use-cases and pain points.
This is why the revolution is happening at the grassroots level. Knowledge workers can use GenAI to accelerate specific tasks but still rely on their own expertise to ensure quality. It’s not plug-and-play autonomy: it’s man-machine collaboration.
At TRUE, an internal survey revealed that about two thirds of our employees were using some form of GenAI (like ChatGPT) to boost their productivity by 10%-30%. The degree of use and productivity gained from GenAI seems to vary by discipline, with areas like marketing and software engineering at the higher end of that spectrum. Before the survey, we estimated that only about one third of our employees were using GenAI tools to boost productivity. We had little insight into the extent of productivity gain that some employees were experiencing.
The Gap Between AI and Human Intelligence
Despite its rapid evolution, GenAI still lacks true human intelligence. It doesn’t reason or understand in the way people do, have human intuition, or common sense. It can generate a thank-you note or summarize a policy document, but when asked to operate autonomously in complex domains, it far too frequently falters.
In chess, GenAI looks to historical patterns. In conversation, it predicts likely next words and phrases. It is a master of pattern recognition, not deep understanding.
The implications are clear: for simple tasks with human oversight, GenAI is transformative. For complex, autonomous systems, it remains risky business without added guardrails.
Trust and Verification in Autonomous Systems
For knowledge worker automation to scale, particularly in regulated industries like mortgage lending, systems must be verifiably correct, explainable, and auditable. That means:
- AI systems must know when they’re right – and when they’re not.
- Humans must be able to review, validate, and sign off.
Explainability, traceability, and control aren’t just nice-to-haves. They’re essential for trust, compliance, and safe deployment.
There’s also a concept of vertically-focused AI that has the potential to work in the background without human oversight and review. These would usually apply to the more tedious, time-consuming tasks where there also exist mechanisms for provable correctness – where the system can demonstrate using blended orthogonal methods that the data and assertions are correct. In this case, the AI-based process elevates from a “virtual assistant” to essentially a “master” of an important tedious task, able to operate standalone. Adoption and ROI for vertically-focused autonomous AI is usually rapid as there is virtually no requirement to retrain the workforce or modify current processes.
Why Mortgage Lending Is Ripe for Disruption
All of this has special relevance to home lending – a process still plagued by manual workflows, slow decisioning cycles, and ever-increasing origination costs.
Transformer-based LLMs and GenAI technology are starting to change that. In the near future, we’ll expect to see:
- Loan Prequalification in minutes, not hours/days
- Intelligent underwriting in virtual real-time, prepping cases for human review
- Automated QC and compliance checks, layered with human validation
- Loan decisioning in days, not weeks
But this won’t necessarily come from replacing people. It will come from giving them smarter, enabling tools – tools that automatically convert documents to digital data, sync all data sources, eliminate repetition, flag exceptions, and handle the mundane.
A Bottom-Up Revolution That’s Just Beginning
Knowledge worker automation hasn’t failed – it just took longer to arrive than expected. And now that it’s here, it’s not starting in corporate boardrooms. It’s starting on desktops and in inboxes, from the ground up.
For forward-thinking companies, this is the moment to get ahead of the curve. Those that seize this moment will find themselves in the near future operating more efficiently, unlocking faster loan underwriting and decisioning, powered by greater automation and reduced reliance on manual checks. Those who wait risk watching from the sidelines, losing both clients and market share.
This is the new industrial revolution for knowledge work. And it’s already underway.
Join the conversation!
Subscribe to our LinkedIn newsletter and look out for future blogs, where we’ll discuss how these AI solutions are transforming document processing, risk management, and overall efficiency in the mortgage industry. Join us to learn about the future of AI in home lending.