Trusted data is the “lifeblood” for mortgage originators, insurers, sellers, buyers, and servicers. It is defined as digital data that is supported by documents or trusted third party sources to define and ensure the quality of the loan package. All decisioning about the loan package depend upon the quality of this digital data. The axiom “garbage in, garbage out” directly applies here. Hence, the term Trusted Lending Data.
The challenge for mortgage companies is that most of the critical data is contained in PDF documents and images provided by borrowers or third parties. While there is increased use of direct digital data (DU validation services for example), PDFs remain the largest and most cost effective source of the data required to support the loan package. And few mortgage industry sages are predicting that this situation will not change much in the near to medium term future.
(Human in the Loop) HITL Approaches to Trusted Data
To obtain trusted data from PDFs, the mortgage industry spends billions of dollars per year to deploy humans (employees, contractors, or through outsourcing to offshore BPOs). Most mortgage lenders have used this approach for several decades. More recently, these labor-intensive approaches have been supplemented with OCR solutions. OCR is a technology that “scrapes” the data from the PDFs to create a digital representation that can be edited. Humans then review the digital data against the original PDF for correctness and make edits as needed. The more effective the OCR is the less work for the humans to correct. OCR has improved over the years, especially for highly structured forms like tax forms and standardized application forms. Even so, the image quality matters a great deal for OCR to be effective.
In the last decade, AI technology has emerged to dramatically improve the accuracy and applicability of OCR. Computer Vision, machine learning, and generative AI (large language models – LLMs) are now used to help machines recognize (without human involvement) broad classes of semi-structured and unstructured document types and all their variants. Once the document is recognized (for example a W2) by a machine, document specific parsers and other approaches are deployed to set up the OCR extraction tool to achieve much higher accuracy across a broad set of documents.
Before AI, OCR was 70-80% accurate. Supplemented by AI, OCR is 90-100% accurate today. The range of accuracy depends upon the AI technology provider and how complete their specific solution is. Solutions that combine AI/OCR technology with humans to perfect that data are called HITL solutions (humans in the loop). An HITL solution with average or partial AI technology may provide 90% accuracy. A solution, with a very advanced processing pipeline of AI technology, will deliver accuracy exceeding human level accuracy, including >99% accuracy.
The Importance of Machine Accuracy
If a mortgage company is processing 2000 loan applications per month, and extracting 100 fields per loan from all the documents provided, this generates 200,000 extracted fields per month. An AI/OCR solution that yields 90% accuracy requires 20,000 fields per month to be corrected. But it’s worse than that. Typically, a 90% accurate AI solution will be confident with about 75% of all fields and ask humans to review the rest. Using that confidence threshold there are now 50,000 fields to review, of which 90% are accurate but still require review. Even the process of correcting a few data fields on a 5 page document will invariably end up requiring a review of the whole document An average time of 1 minute to review and correct such data fields, results in 50,000 minutes of human time for 2000 loans per month This requires a full-time staff of 5-6 to do this work. Each loan will take 25 minutes (25 of the 100 fields left to review at 1 minute per field) to run extraction, and then review and correct as required, slowing all decisioning processes that require trusted data.
If the AI accuracy is 99% then only 2000 fields are left for review. But the AI will require that 5% of all fields need review and 99% of the reviewed fields will be correct. In this case, for 2000 loans, 10,000 fields need review each month. This can be done by 1 full-time person. Equally important, each loan will only require 5 fields for review (5% of 100) resulting in loan extraction processing in only 5 minutes. With AI technology, the review and correction of fields can be further surgically targeted to quickly take the reviewer to the specific field in the documents avoiding unnecessary clicks needed to first open the processed document batch, then the document and then the appropriate fields in the document
This differences in 99% AI versus 90% AI is summarized in the following chart:
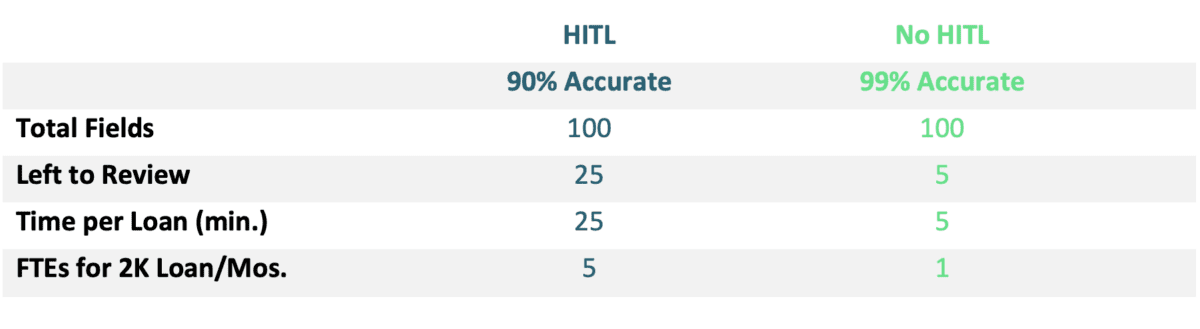
The Highest Cost Area for Trusted Data
Unfortunately getting the data corrected is not even halfway towards obtaining trusted data. Ensuring that the data extracted from a paystub is accurate to the original PDF paystub, is an important first step. But how do you know that the address, borrower names, social security numbers, property address, asset data, income data, etc. are valid entries and consistent across the entire loan file? There are dozens of documents and data sources that contain the same fields. Any discrepancies (differences in fields that should be identical) need to be discovered and corrected for this digital data to become trusted data. As an example, an underwriter cannot move forward to approve a loan if the property address is correct on 4 documents but wrong on another. To correct this, the underwriter or the processer will need to look at all documents to verify all the relevant fields, and in some cases go back to the borrower for clarification. Now multiply this effort by hundreds of fields and dozens of documents per loan to gauge the extent of the effort to verify the data.
Getting a “clean loan file” with fully trusted data is one of the most expensive and time-consuming stages in loan manufacturing. In fact, the cost to verify the loan file because of all the “stare and compare” required, can be triple the cost to get the PDF data into accurate digital form. Again, using the example above, verifying the loan data will take an additional 75 minutes (25 minutes to extract/correct and 3x that for verify) for the 100 fields in the example. During those 75 minutes, the processor will open up as many as 40 documents, determine which of the 100 fields are on each document, and then locate and compare the data for consistency.
AI to the Rescue Again
Once again intelligent solutions that leverage advanced AI technology can automate the massive amount of staring and comparing required to fully verify a loan file. TRUE is an example of a complete solution that uses a trusted lending data pipeline to (1) almost entirely reduce the HITL costs to get accurate digital data from PDFs and (2) fully automate data verification. TRUE’s data pipeline is shown here:
TRUE’s Proprietary Trusted Lending Data Pipeline
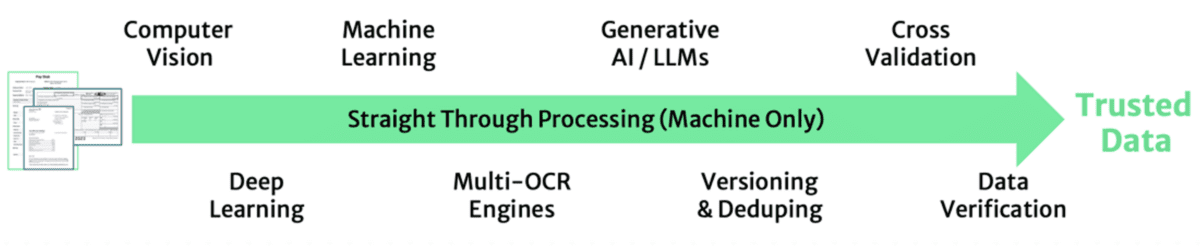
TRUE Results: Machine-only AI Assistants generate more accurate Trusted Data at a 10x faster rate than humans today.
TRUE’s cross validation and data verification engines in the pipeline automatically compares the accurate extracted data with other independent sources for the same data. When a match is found, the data is considered cross-validated and human review is not required. Data Verification adds a final crucial step by automatically opening up every document and comparing all data fields for consistency across the entire loan file and MISMO record for the loan. This 360 degree view of the data is unique to TRUE and establishes the trusted data certification that lending decisioning requires. All of these verifications happen continuously helping uncover discrepancies early on in the process, the minute a document is uploaded, facilitating immediate remediation such as asking the borrower to immediately provide an updated document, reducing the time spent in chasing these down at the time of underwriting or closing.
Trusted Data Case Study
In a recent case study, applying this pipeline to a loan package that required 100 fields to be extracted yielded a 10X improvement in generating trusted data when automation is applied to both stages of trusted data generation.

Extract & Correct: Using AI/OCR solutions to extract data from PDFs, humans (HITL solutions) then used to compare and correct extracted data to original PDF
Verify & Validate: Checking to see if the extracted data is consistent with all other document and data sources (i.e. is the property address valid and is it the same throughout the entire loan record?) requires opening up all docs and comparing all fields in LOS for validity and consistency.
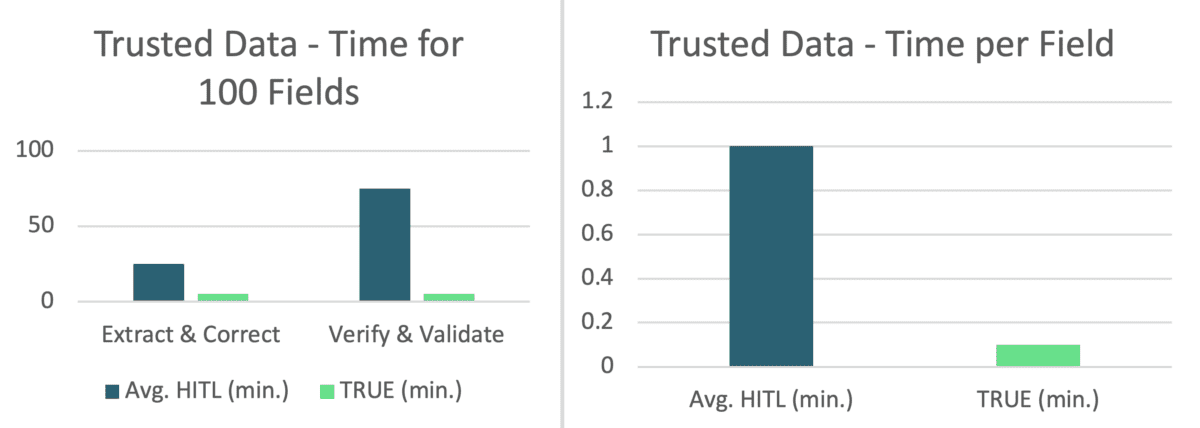
Obtaining trusted data from PDFs is one of the most critical areas for mortgage companies to invest in to improve costs, efficiency, and competitiveness. A complete solution requires automating both the digital data extraction (to reduce the HITL costs and time) and the data verification stage (to eliminate the manual “stare and compare”). TRUE is the only solution that automates both areas resulting in a 10x improvement over other AI/OCR HITL solutions available today.